Use Case
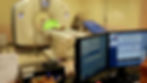
Radiology Report Generation through Generative AI by Gennet.AI
Challenge:
Radiology Report Bottlenecks
​
In the realm of medical imaging, radiology reports are a critical component of patient care, providing essential diagnostic information. However, the generation of accurate and timely radiology reports has long been a bottleneck in healthcare. Radiologists are often overwhelmed with a high volume of images to interpret, leading to delays in report generation. Additionally, variability in reporting styles and the potential for human error can affect the quality of reports, impacting patient care and clinical decision-making.
Solution:
Generative AI for Radiology Report Generation
​
Gennet.AI, a pioneering product company in medical generative artificial intelligence, has risen to address these challenges with a groundbreaking solution: Generative AI for radiology report generation.
​
-
Automated Report Generation: Gennet.AI's AI system is designed to automatically generate radiology reports from medical images. This alleviates the burden on radiologists, allowing them to focus on image interpretation and patient care.
-
Consistency and Standardization: The system ensures consistent reporting by adhering to established guidelines and clinical protocols, eliminating variations in reporting styles. This standardization enhances the quality and reliability of radiology reports.
-
Efficiency Enhancement: By automating the reporting process, Gennet.AI significantly reduces the time required to generate reports, facilitating quicker diagnoses and treatment decisions.
Implementation:
A Seamless Workflow Integration
​
Gennet.AI's implementation of Generative AI for radiology report generation involves several key components:
​
-
Data Integration: The system integrates with hospital or clinic picture archiving and communication systems (PACS) to access medical images. It can handle a wide range of image modalities, including X-rays, MRIs, CT scans, and ultrasounds.
-
Generative AI Models: Gennet.AI employs deep learning models, such as convolutional neural networks (CNNs) and recurrent neural networks (RNNs), to extract information from medical images and convert it into textual reports.
-
Natural Language Processing (NLP): Advanced NLP techniques are applied to ensure that the generated reports are coherent, clinically meaningful, and contextually relevant.
-
Customization: The system allows for customization to accommodate specific reporting preferences and requirements of healthcare institutions.
Impact:
Advancing Patient Care and Radiology Efficiency through Generative AI
​
The introduction of Generative AI into radiology report generation has profound impacts on healthcare delivery:
-
Swift Report Turnaround: Radiologists can now produce reports rapidly, reducing patient wait times and enabling faster treatment planning.
-
Enhanced Quality: Standardized reports ensure consistency in diagnostic communication, leading to improved patient outcomes and reduced chances of misinterpretation.
-
Reduced Workload: Radiologists experience reduced workloads related to report generation, allowing them to dedicate more time to image analysis and patient interaction.
-
Cost Savings: Efficiency gains translate into cost savings for healthcare institutions as they optimize resource allocation.
Conclusion:
A Paradigm Shift in Radiology Reporting
​
Gennet.AI's innovative use of Generative AI in radiology report generation represents a paradigm shift in the field of medical imaging. By automating and standardizing the reporting process, they have not only improved the efficiency of radiology departments but have also elevated the quality of patient care. As Gennet.AI continues to refine its AI-driven solution and expand its capabilities, the future of radiology holds the promise of faster diagnoses, reduced errors, and ultimately, better health outcomes for patients worldwide.
.png)